“The only rules are the ones dictated by the laws of physics. Everything else is a recommendation.” — Walter Isaacson/Elon Musk
We live in a spreadsheet society. Columns of Categories of People and Rows of Wants, Needs, and Desires. This model is too simplistic. What is needed, and Big Data can provide, is a more sophisticated approach.
This spreadsheet approach was shown in the recent US elections to be detrimental to the party mainly depending on this view of society. How many times did we hear pollsters opine that X percent of a certain population was voting for one candidate vs. the other? The categories of people were divided along traditional lines. This whole model was shattered on November 2, 2024, and likely some providers of data in this format may no longer be in business for the next cycle.
However, this belief system is not limited to the political class. We all can fall into this stereotype.
For decades we have been taught that data categories can be captured under a Normal Distribution (Bell) Curve of a category and row of interest, i.e., the distribution of the height of a class of male senior high school students or SAT scores. Another more relevant example, retailers’ pertinacious obsession with the 18–34-year-old group.
These are fairly simple models, and in this Blogger’s opinion, this representation rarely works anymore, if it ever did. For example, the resulting retail sector inventory overages as a result of dependencies on this gross data model are not an effective return on shareholder value. Other recent missteps based on faulty interpretation of the customer/prospect base include the Bud Lite advertising fiasco and the Target marketing failure.
It is ok to make marketing mistakes, that is going to happen. The problem with these two (and other) campaigns is the analysis of risk, return, etc. was likely shallow or mathematically primitive.
There are ways to appeal to new consumers without alienating a large existing base. It’s all in the big numbers.
There are several validated models of human behavior and we will discuss two of them herein, Maslow’s Hierarchy and the Relationships, Behaviors, Conditions model. Some readers may prefer others, and they will most likely work within this construct as well.
Maslow’s Hierarchy of Needs
This perspective on human needs and subsequent behaviors dates back to the 1940s. As shown, it consists of five steps from survival to the ultimate Self Actualization. Note that we adapt this model in two ways. First, we view this as a growth process as opposed to five discrete steps. Additionally, the bottom two lower ranges focus mostly on the physical issues we all face. Fundamental survival and security both physically, mentally, emotionally and spiritually. Once we have attained, sustained, and believe, we are ready to transition towards an end game, “the realization or fulfillment of one’s talents and potentialities, especially considered as a drive or need present in everyone.”
Next, we will discuss how we can use this and the RBC solutions to realize specific and measurable value that changes this dynamic.
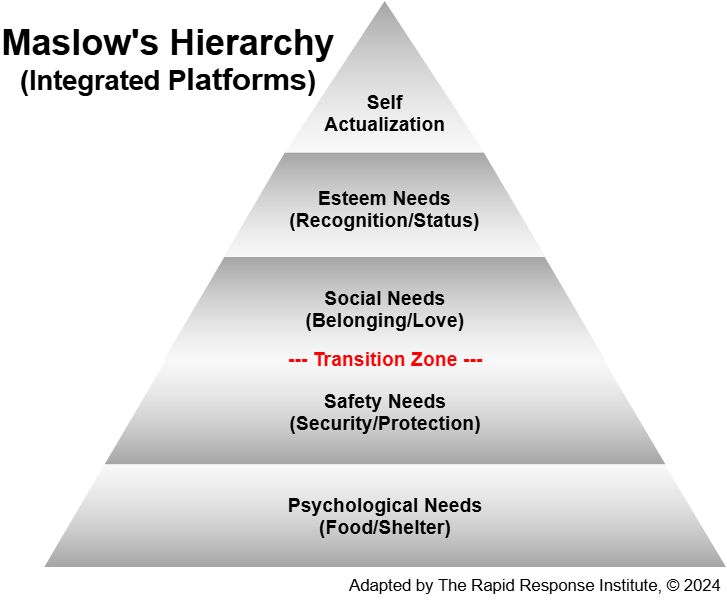
The color gradient is meant to reflect that this journey is a process and often each field begins slowly before accelerating into the next range.
Another perspective of each the Five Platforms consists of a number of smaller steps, which when assessed using Integral Calculus, generates a continuum.
Relationships, Behaviors, and Conditions (RBC)
The following is taken from our Cross-Cultural Online Game site:
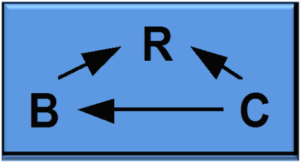
The RELATIONSHIPS, BEHAVIORS, and CONDITIONS (RBC) model was originally developed to address issues around cross cultural (international) negotiation processes. As shown in the figure, Relationships are the focal point of this perspective, reflecting commonality of interest, balance of power and trust as well as intensity of expressed conflict. Behaviors in this model is defined as a broad term including multi-dimensions and intentional as well as unintentional. Finally, Conditions are defined as active and including circumstances, capabilities and skills of the parties, culture, and the environment. Of course, Time is a variable in this model as well.
Moreover, we have defined Behavioral Economics as “the decision-making model that incorporates societal, cultural, emotions and other human biases into the process as opposed to the classic rational economic actor.
One key feature of the R B C Framework is its emphasis on interactive relationships while providing an environment for multiple levels of behavioral analysis. This makes it a useful tool to better understand the new Big Data/AI processes currently unfolding.
The following graphic is a derivative of our Cross-Cultural Interactions model. It is a peer reviewed model and is a very good way to calibrate interactions. Additional information is available on the above link.
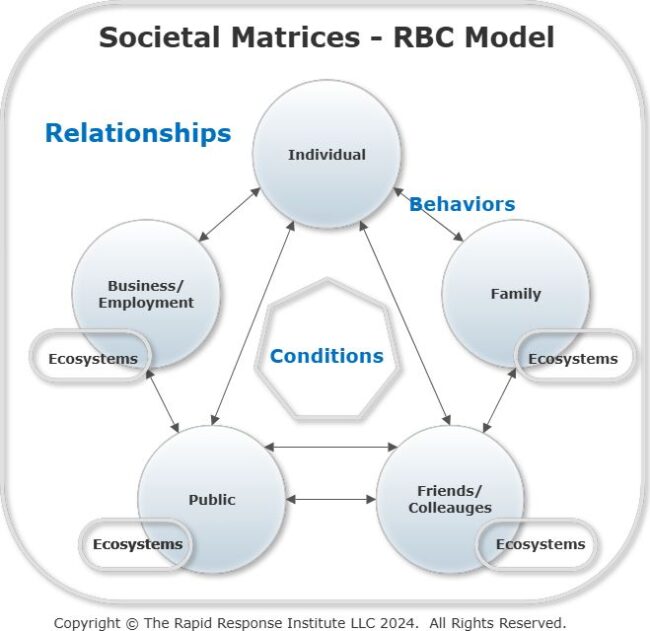
This author has long believed that we do not live in a linear or mathematically deterministic world. A strong belief in stochastic, matrices drive much of my thinking. This is reflected in the figure on the left. The RBC model is the foundation for the five parties and their collective Behaviors. The resulting Relationships range from one-on-one to small groups or in some cases a wide and varied constituency.
Note that there is an Ecosystem, (something, such as a network of businesses, considered to resemble an ecological ecosystem especially because of its complex interdependent parts) associated with each entity other that the individuals’ who are the other four.
This is a wide array of influences on any given individual. Not easily measured, linearly!
Scientific Method
Most readers are familiar with how science has been brought into bolster the position of pundits/advocates of policy, especially related to Covid-19. The word is tossed around casually, as if most who use it know what they are talking about. Spoiler Alert–Most Do Not! This includes some with advanced technical or medical bona fides.
Most think that by using the word ‘science’ this assessment process is out of their wheelhouse. Previously, we argued that there is a layperson’s approach that works fine for most of our daily needs. Details can be found on our Blog, They Blinded Me with Science.
For space reasons, this (guidelines) model will not be repeated here but check it out, it is a short read.
The late Nobel Laureate, Richard Feynman has a brief and layperson-oriented presentation on the Scientific Method. He is an excellent teacher, and this clip is informative as well as entertaining.
“If it disagrees with experiment, its wrong”—Richard Feynman
Human Input Still Needed
To be clear, pollsters and marketers will still need to ask questions that will also help structure the model, but the processes posited herein can change the weighting processes driving toward equilibrium and Pareto Optimality (Pareto efficiency implies that resources are allocated in the most economically efficient manner but does not imply equality or fairness) in the final analysis. In other words, higher quality results.
These numbers can also be seen as ‘first value’ in a simulation. Another way to look at this is to the logic the Turing (Bombe) Machine of World War II. Not setting a number as itself.
Then AI can take the model to the next level and provide pollsters/marketers with real, modern solutions. Finally, other uses will most likely be derivatives of this solution.
The Data Management Problem
We have identified a general problem as well as three valid and reliable theories. This is only a nice discussion without relevance with Valid, Reliable, and Timely (VRT) data. Data Management has been a problem as long as there has been data, stone, paper or electronic. It has always been an issue. “Herman Hollerith is given credit for adapting the punch cards used for weaving looms to act as the memory for a mechanical tabulating machine, in 1890.” Much later (circa 1950s) digital database management schemas emerged. Today, we are flooded by data of all types including telemetry and deliberately false data/information.
How can this be effectively managed and how can an executive without a technology background survive much less thrive in such an environment?
For decades, this author has been involved in almost all computer system development from the yellow punch tape of the 1960s until today. There have been several constants over that period.
One of the biggest chronic challenges has been database management; from the acquisition of data through its life cycle until achieve. An outcome of poor data management has been decisions that have even led to the demise of firms, along with tens of thousands (if not more) of suboptimal decisions at the expense of shareholder value (bottom line/stock price). What makes the current crop of Big Data/Artificial Intelligence advocates any smarter than those who came before? Nothing! Unless some things are changed.
Our forthcoming book to be published by CRC Press in 2025, Navigating the Data Minefields: Management’s Guide to Better Decision-Making is a book for the non-IT executive who is faced with making major technology decisions as firms acquire advanced technologies such as Artificial Intelligence (AI). The following and other managerial action items are developed in detail.
- How to determine the quality of the data and its relevance to the decision-making processes.
- Current Challenges and Trends in Big Data and associated applications.
- Proposed organization structure such as High Reliability Organization and an understanding of Human Factors to fully realize the full and measurable economic value from these technologies.
- A full set of Risk Mitigation and a Governance model including Disaster Recovery and Cyber Security.
- How these technologies are used in Operations, a proposed Management System as well as numerous Case Studies across a number of industries and types of problems.
The challenge of managing this suite of emerging AI/Big Data is daunting and one that cannot be dodged or delegated. How organizations respond can be the difference between success or the destruction of shareholder value.
It’s The Data Stupid
Our pollsters are collecting and analyzing data that are self-reported.
- A questionnaire is developed which may or may not reflect bias.
- These questions are posed to potential respondents (phone and otherwise) who may detect a voice tone, and/or the subject may intentionally lie or mislead.
- Finally, who answers the phone these days? This skews the data sample and perhaps badly.
Data collected in this manner is not subject to rigor and most likely wrong or skewed. Finally, data collected in this manner is not suitable for the new Big Data Analytic models.
One Proposed Better Way
There are probably several much better ways to address political and marketing problems. While no data source is perfect, we posit the following way to use the US Census data. We can treat it as a function of columns and rows yet apply sophistical data analysis algorithms.
Categories
Rows
Wants
For purposes of this model, we define Want as something that an individual might seek as part of normal life, i.e., and ice cream cone.
Needs
Those fundamentals of life, especially as defined in Maslow’s Hierarchy, platforms one and two.
Desires
Sources of Wants, Needs, and Desires
We have suggested that a starting point for Categories is the very large US Census database. No such singular source exists for these behaviors and there is an overlap in definitions. This list gives readers a starting point. In no particular order, this non-comprehensive includes:
- A Theory of Motivation proposed by (the late) Steven Reiss, Psychology and Psychiatry professor emeritus at Ohio State University in Ohio, USA.
- Human Wants: Meaning, Classification and Characteristics: A listing of economic and non-economic wants.
- The Four Stages of Desire: From Everything to One Thing
- 30+ Human Needs: A Comprehensive List — maps to Maslow’s Hierarchy
- The Ultimate List of 100 Life Goals To Achieve Before You Die
The list goes on, but readers get the point. A large row of behaviors will help develop a robust model.
And The Answer Is
While we know that the solution to the political pollster and/or marketing manager is not a definitive answer, we can do better than we are. We know how to management data in this environment and we have models for assessing and making decisions based on results. As we move from simple, small data set, linear models to robust Big Data Analysis, we need to consider a few additional action items.
Scientific Method
Do not forget to use this methodology to define and refine your problem statement.
Model Limits
- While we are using a significant number of independent and dependent variables, we are not trying to solve world hunger. Therefore, we need to put limits on (Bound) the model. This is an age-old problem we first addressed in 2015, Bounding the Boundless.
- From a scientific perspective, “Across all science, modelling is our most powerful tool, as models let us focus on the few details that matter most, leaving many others aside. Models also help reveal the typically far-from-intuitive consequences when multiple causal factors act in combination.”
- Additionally, “Getting AI/ML/DL systems to work has been one of the biggest leaps in technology in recent years, but understanding how to control and optimize them as they adapt isn’t nearly as far along. These systems are generally opaque if a problem develops in the field. There is little or no visibility into how algorithms are utilized, or how weights that determine their behavior will change with a particular use case or interactions with other technology.”
- Moreover, “the European Union this week (2021) issued guidelines for AI — specifically including ML and automated decision-making systems — limiting the ability of these systems to act autonomously, requiring ‘secure and reliable systems software,’ and requiring mechanisms for ensuring responsibility and accountability for AI systems and their outcomes.”
Diminishing Returns
Since we are not boiling the ocean, at some point continuing with the model will result reduced performance. According to Economists, “The law of diminishing returns says that, if you keep increasing one factor in the production of goods (such as your workforce) while keeping all other factors the same, you’ll reach a point beyond which additional increases will result in a progressive decline in output. In other words, there’s a point when adding more inputs will begin to hamper the production process.”
“Data is just a way of codifying information. Any data gathered should be relevant to a problem, otherwise useless data clouds the results of a query. If there are too many degrees of freedom, you are begging for a spurious correlation.”
Need for a Data Scientist
This approach may require the use of a professional data scientist to realize a valid and reliable outcome. “A data scientist is an analytics professional who is responsible for collecting, analyzing and interpreting data to help drive decision-making in an organization. The data scientist role combines elements of several traditional and technical jobs, including mathematician, scientist, statistician and computer programmer. It involves the use of advanced analytics techniques, such as machine learning and predictive modeling, along with the application of scientific principles. As part of data science initiatives, data scientists often must work with large amounts of data to develop and test hypotheses, make inferences and analyze things such as customer and market trends, financial risks, cybersecurity threats, stock trades, equipment maintenance needs and medical conditions.”
More
This is not a definitive list and is provided to give readers an understanding of what it will take to move forward with the Big Data Analysis model. Appropriate research and problem definition may reveal additional or fewer requirements.
Demise of Linear
The Linear broadcast approach to selling or changing minds is not the relevant delivery vehicle for new micro-data analysis. Directed notifications is better although practice this with care. This last election cycle, I received a text, words to the effect, “can we count of your vote for X?” I was very much against X and no reason was offered to help me change my mind. Get with the program and don’t be so lazy developing your spiel or message.
Elon Musk’s ‘Algorithm’
This is an interesting approach to problem solving that can have relevance to the issue discussed herein. Can we reduce complexity without losing data fidelity, “The accuracy, completeness, consistency, and timeliness of data. In other words, it’s the degree to which data can be trusted to be accurate and reliable.”
Question Every Requirement
Delete Any Part or Process You Can
Simplify and Optimize
Accelerate Cycle Time
Automate
Readers may ask, how does this fit in this discussion? We bring this to your attention because it may help identify and bound the problem to be solved.
Also, perhaps of some value is his, “idiot index, which calculated how much more costly a finished product was than the cost of its basic materials. If a product had a high idiot index, its cost could be reduced significantly by devising more efficient manufacturing techniques.” A survey, analysis, etc. are all products and may benefit from this index model.
Final Thoughts
Most financial professionals will tell you that spreadsheets must foot (the sum of all rows must equal the sum of all columns). Can societies or multiple societies foot their columns and rows? Most likely not and if that is the case, data from this model is not valid or reliable.
Most likely, this type of data will take the form of a Scatter Diagram (without correlation?) with some clumps or areas of intensity of a specific category or group of categories.
This may not be an approach that many will take; however, it is clear that the original premise of this piece, that we live in a spreadsheet society is no longer appropriate. “If you always do what you always did, you will always get what you always got.” ― Albert Einstein. It will be interesting to see how future polling is conducted.
Non-Linear Speed Ahead
Sometimes pieces like this summarize a conclusion. In the high-pressure environment, we are not concluding anything. We are carving a way forward–A Growth Model of the Data Economy.
As of this writing the incoming US administration appears to be moving at hyper speed across a broad front. Moreover, AI in particular exploding. Those interested in keeping up may want to to Subscribe | Generative’s AI Newsletter (not an endorsement but seems be a good daily source of information). Mostly, just keeping up will not be enough, if top quartile success is the goal.
It will be interesting to see the role the family of Artificial Intelligence (AI) software solutions play in this field going forward.
Micro-targeting is certainly a place for Big Data to shine. That said the problem to be solved and the confidence in the validity and reliability of the data must ascertained.
Society faces some real challenges with how we manage, analyze and decide using data. This is but one example where old methods no longer produce valid and reliable results. How will you and your organization go forward?
For More Information
Please note, RRI does not endorse or advocate the links to any third-party materials herein. They are provided for education and entertainment only.
See our Economic Value Proposition Matrix® (EVPM) for additional information and a free version to build your own EVPM.
The author’s credentials in this field are available on his LinkedIn page. Moreover, Dr. Shemwell is a coauthor of the recently published book, “Smart Manufacturing: Integrating Transformational Technologies for Competitiveness and Sustainability.” His focus is on Operational Technologies.
We are also pleased to announce our forthcoming book to be published by CRC Press in 2025, Navigating the Data Minefields: Management’s Guide to Better Decision-Making. This is a book for the non-IT executive who is faced with making major technology decisions as firms acquire advanced technologies such as Artificial Intelligence (AI).
“People fail to get along because they fear each other; they fear each other because they don’t know each other; they don’t know each other because they have not communicated with each other.” (Martin Luther King speech at Cornell College, 1962). For more information on Cross Cultural Engagement, check out our Cross Cultural Serious Game. You can contact this author as well.
For more details regarding climate change models, check out Bjorn Lomborg ands his latest book, False Alarm: How Climate Change Panic Costs Us Trillions, Hurts the Poor, and Fails to Fix the Planet.
Regarding the economics of Climate Change, check out our blog, Crippling Green.
For those start-up firms addressing energy (including renewables) challenges, the author can put you in touch with Global Energy Mentors which provide no-cost mentoring services from energy experts. If interested, check it out and give me a shout.
Copyright © 2024 The Rapid Response Institute LLC. All rights reserved.